Data migration plan: verifying prerequisites for Data Migration
Data migration is the set of processes required to transfer data from a legacy data management system to a new system. In terms of product information management, these processes require profiling, selecting, preparing, extracting and transforming before loading to the new system and carrying out post-migration standards verification.
What is a data migration plan?
The migration plan defines the methodology governing principles for ensuring the move from old to new product data management system takes place in a smooth and organised manner. The strategy must be built to prevent a badly-designed and run migration project from creating more problems than solutions (such as moving bad data across to the new system).
What are the key data migration steps?
There are three principal phases in data migration:
Initiation, planning and designing, where the project team documents the strategy, scope and governance of the migration.
The build is made up of the tasks needed to transfer source data from the existing to the new system
The cutover involves all work required to complete project, principally, the final load, validation and sign-off on the final iteration
Let’s look in more detail about what these steps entail in terms of strategy and planning.
Key Stages in a Data Migration Strategy
A typical set of tasks for building a data migration plan include the following:
- Discovery phase - to evaluate data contained in the source system. It is essential to have in-depth knowledge and understanding of what is to be migrated, and how it is to fit into the target system.
- Define and Design the Migration - This includes migration schedules and priorities, settings for backup and replication, capacity planning, and prioritisation by data value. It also covers possible changes to data models, as it may be necessary to change the model if, for example, the company is moving from an on-premise data warehouse to a cloud-based data warehouse, or if moving from a relational data infrastructure to a blend of structured and unstructured data.
- Migration Solution build - The data migration project is as much a business process as a set of technology-driven processes. If end-users are involved closely from day one. It is much easier to build a solution based on full knowledge of data rules, definitions, compliance, and priorities. That informs the modelling requirements which emerge. Live Testing - System testing with all data should be carried out – those which are used in the legacy system, as well as the new data. Additionally, existing functionalities should be verified alongside the new or modified functionalities.
- Cutover - A successful go-live takes months and months of hard work. The literature suggests the mantra is ‘test, test, test’! Many organisations have trial runs where stakeholders go through every and any scenario to assess worst-case scenarios. That way, should any crisis occur, a calm and information-driven approach can be taken. Migration Audit - This involves forensic checking of the operational capacity and emerging issues of the system after a period of live operations.
Rollback plan for data migration
In a nutshell, a rollback plan (or recovery plan) is put in place so that if any issues occur during the migration, the system can be reverted to its last known ‘good’ state.
At all stages of the project, backups and rollback planning is essential to apply whatever ongoing fixes are needed. An example of rollback implementation is that of establishing checkpoints at certain phases of the migration, to run periodic assessments on the data. If a roll-back is required during transition, the necessary backups and deployments can be made, without having to halt the project for ‘repairs’. The absence of a rollback plan adds extra work and time to the process and will almost certainly cause avoidable disruptions in the new system after deployment.
Cutover plan for data migration
The cutover refers to the transition from one phase of a migration project to the next. It has to be a carefully choreographed enterprise, involving project manager, system administrators, storage administrators and data owners. Stakeholders should fully understand each step of the cutover process, step by step and item by item, and the completion of each step must be formally signed off by all concerned.
Optimal cutover plans usually have a contingency plan which closely documents fallback protocols in case of failure. A cutover is intrinsically disruptive, so a key aim must be to minimise any problems.
There are essentially 3 options for the cutover strategy – big bang, incremental or parallel.
Big bang
This involves building the data migration architecture and transferring the data to the target system all at once. A window of opportunity is needed to carry this out successfully, as it will inevitably mean a period of downtime for company operations.
Incremental (sometimes known as ‘trickle’)
This works best for companies with geographical or functional divisions, as the approach is more piecemeal. Data can be moved more slowly, over a longer period. This approach reduces the risk factor, as it is generally much easier to roll back a smaller subset of data in the case of problems.
Parallel
This involves transferring the data to the target system while ensuring that the data in each system remains up to date. The two systems can run in parallel over a period of time to allow the company to fully validate and sign-off on the new platform. However, this approach is expensive (maintaining two systems simultaneously) and can also be technologically complex.
The data migration testing process
There are typically three stages:
Pre-migration testing: this ensures the data schema is consistent by testing the fields linking source and target records to verify definitive mapping among the records
Data cleansing: this can be a major undertaking, as it requires an understanding of all error types – empty fields, overlong character strings, or incorrect entries. Clarity is also needed to maintain data linkages: altering one table may damage the data in another. Finally, data interrogation is carried out on all datasets being migrated, to identify and find data with the previously established error categories.
Post-migration testing: This takes place to check the consistency between validation of the user interface and of the database. Any inconsistencies are noted as defects.
It is also important to ensure data is ’locked’ – that is, while data is being written, multiple users are not permitted to access the same new record in the database.
Data migration test sample
There are certain migration tests to identify issues during the migration. Two examples are:
Rollback Testing (verifying the rollback is in place) exists in the case of a migration failure at any time during migration, so that the legacy system can resume operations rapidly with minimal impact on users.
Backward Compatibility Testing checks that the new system is compatible with the legacy one (over at least 2 previous versions) and that it will work smoothly with those two versions.
Of course, Failure to program test samples runs the risk of damaged migration, with missing, inconsistent, or corrupt files. This impacts not only on the system’s perceived reliability for users, but also its intrinsic value as a business asset.
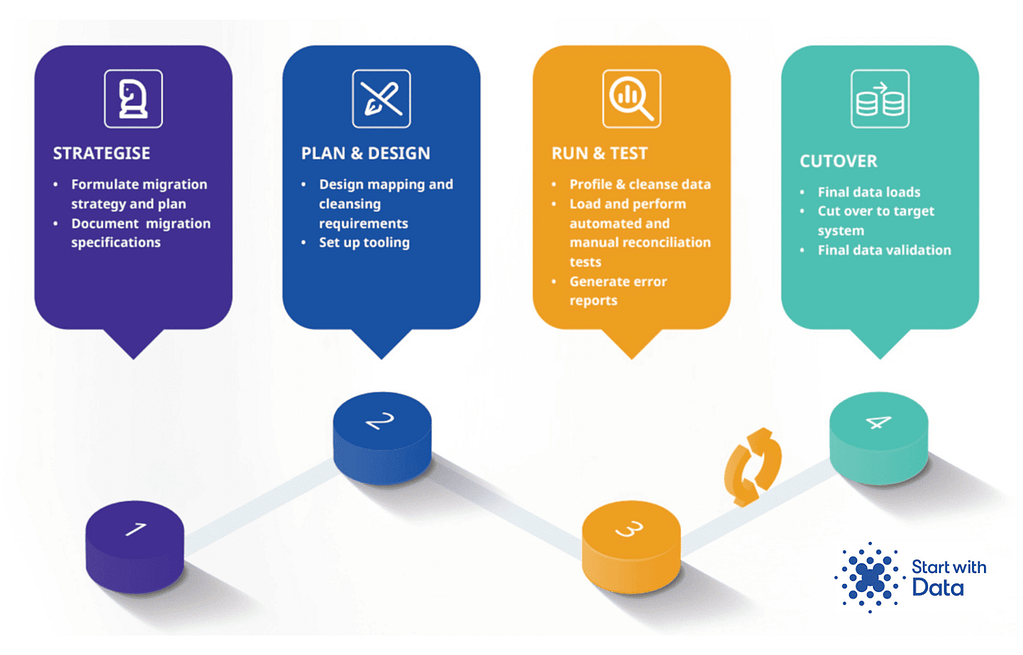
Take the risk out of your data migration with our turn-key data migration approach. Our proven four-step process enables you to transfer data en masse quickly and efficiently – without burdening your internal teams – so, contact us for a conversation about how we can support you with your data migration.
Ready to take the next step in your product data journey?
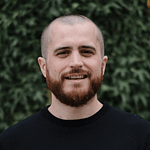
For retailers and distributors
We have a highly experienced team of PIM consultants ready to transform your product information challenges into business benefits – Ben Adams, CEO
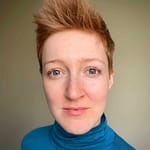
For brands and manufacturers
We can your business compete and thrive on the digital shelf – Beth Parker, Lead Consultant